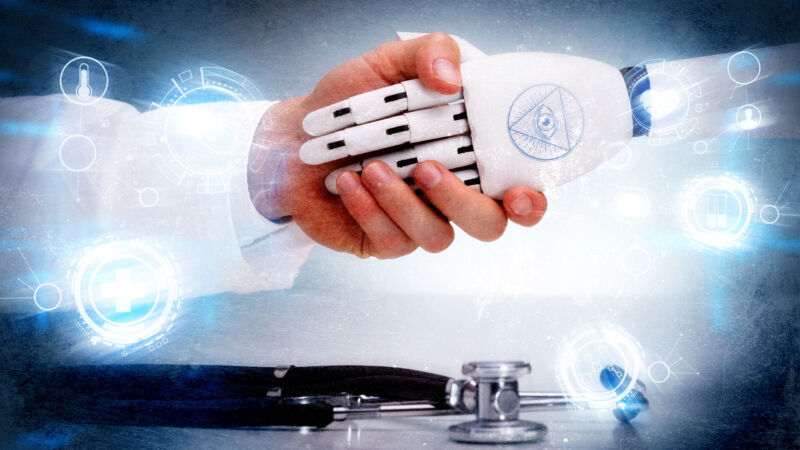
Aurich Lawson | Getty Images
When it comes to artificial intelligence, the hype, hope, and doom are suddenly everywhere. But the turbulent technology has long made waves in healthcare: from IBM Watson’s failed foray into healthcare (and the long-held hope that AI tools could one day beat doctors in detecting cancer on medical images) to the realization problems of algorithmic racial bias.
But behind the public strife of fanfare and failure lies a chaotic reality of rollout that has remained largely untold. For years, healthcare systems and hospitals have struggled with inefficient and, in some cases, doomed attempts to adopt AI tools, according to a new study led by Duke University researchers. The study, posted online as a pre-print, pulls back the curtain on these messy implementations while seeking lessons learned. Amid the revelations of 89 professionals involved in the rollout at 11 healthcare organizations, including Duke Health, Mayo Clinic and Kaiser Permanente, the authors put together a practical framework for healthcare systems to follow as they roll out new AI tools. .
And new AI tools keep coming. Last week, a study in JAMA Internal Medicine found that ChatGPT (version 3.5) decisively beat doctors in providing high-quality, empathetic answers to medical questions people posted on the r/AskDocs subreddit. The superior responses – as judged subjectively by a panel of three physicians with relevant medical expertise – suggest that an AI chatbot like ChatGPT could one day help physicians tackle the growing burden of responding to medical messages delivered through online patient portals. sent.
This is no sinecure. The increase in patient messages is linked to high rates of physician burnout. According to the study’s authors, an effective AI chat tool could not only reduce this exhausting burden – providing relief to physicians and freeing them to focus their efforts elsewhere – but it could also reduce unnecessary office visits, increase patient compliance and encourage adherence to medical guidance, and improve patient health outcomes overall. In addition, better responsiveness to messages could improve patient equity by providing more online support to patients who are less likely to schedule appointments, such as those with mobility issues, work limitations, or fear of medical bills.
AI in reality
That all sounds great, like much of the promise of healthcare AI tools. But there are some major limitations and caveats to the study that make the real potential for this application more difficult than it seems. For starters, the types of questions people ask on a Reddit forum aren’t necessarily representative of the questions they’d ask a doctor they know and (hopefully) trust. And the quality and types of answers volunteer doctors give to random people on the Internet may not match the answers they give their own patients, with whom they have a steady relationship.
But even if the core findings of the study hold up in real doctor-patient interactions through real patient portal messaging systems, there are many more steps to take before a chatbot can achieve its lofty goals, according to the revelations of the Duke-led preprint study.
To save time, the AI tool must be well integrated into a health system’s clinical applications and into each physician’s established workflow. Physicians are likely to need reliable, possibly 24-hour, technical support in case of malfunctions. And doctors should create a balance of trust in the tool — such a balance that they don’t blindly pass AI-generated answers to patients without review, but know they don’t have to spend so much time editing answers that it negates the usefulness of the tool.
And after managing all that, a health system should have an evidence base that the tool works as hoped in their particular health system. That means they should develop systems and metrics to track outcomes, such as physician time management and patient equity, adherence, and health outcomes.
These are tough questions in an already complicated and cumbersome health system. As the preprint researchers note in their introduction:
Based on the Swiss Cheese Model of Pandemic Defense, every layer of the healthcare AI ecosystem currently contains large gaps that make the widespread proliferation of underperforming products inevitable.
The study identified an eight-point framework based on steps in an implementation when decisions are made, whether by an executive, an IT leader or a frontline clinician. The process includes: 1) identifying and prioritizing a problem; 2) identify how AI could potentially help; 3) develop ways to assess the results and successes of an AI; 4) figuring out how to integrate it into existing workflows; 5) validating the safety, efficacy and equity of AI in the healthcare system before clinical use; 6) rolling out the AI tool with communication, training and trust building; 7) supervision; and 8) updating or decommissioning the tool as time passes.