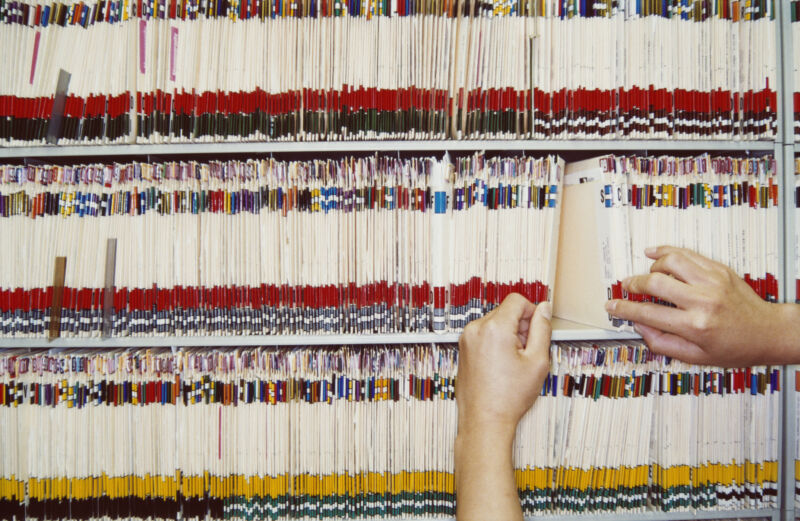
Ten years ago, 12-year-old Rory Staunton dove to a ball during gym class and scraped his arm. He woke up the next day with a 104°F fever, so his parents took him to the pediatrician and eventually to the emergency room. It was just the stomach flu, they were told. Three days later, Rory died of sepsis after bacteria from the scraping infiltrated his blood and caused organ failure.
“How does that happen in a modern society?” said his father, Ciaran Staunton, in a recent interview with Undark.
More than a quarter of a million people die each year in the United States from sepsis, more than a stroke, diabetes or lung cancer. One reason for all this carnage is that sepsis is not well understood, and if not discovered in time, it is essentially a death sentence. Therefore, much research is focused on detecting sepsis early, but the complexity of the disease has plagued existing clinical support systems — electronic tools that use pop-up alerts to improve patient care — with low accuracy and high false alarm rates.
That may soon change. In July, Johns Hopkins researchers published a trio of studies in Nature Medicine and npj Digital Medicine, demonstrating an early warning system that uses artificial intelligence. The system caught 82 percent of sepsis cases and reduced deaths by nearly 20 percent. While AI, in this case machine learning, has long promised to improve healthcare, most of the studies demonstrating its benefits have been conducted on historical data sets. Sources told Undark that, to their knowledge, no AI algorithm has shown large-scale success when used on patients in real time. Suchi Saria, director of the Machine Learning and Health Care Lab at Johns Hopkins University and senior author of the studies, said the novelty of this study is how “AI is being implemented at the bedside, used by thousands of providers, and where we are. see lives saved.”
The Targeted Real-time Early Warning System, or TREWS, scans hospitals’ electronic health records — digital versions of patients’ medical histories — to identify clinical symptoms that predict sepsis, alert health care providers to patients at risk, and facilitate early treatment. By leveraging massive amounts of data, TREWS provides real-time patient insights and a unique level of transparency in its reasoning, according to study co-author and Johns Hopkins internist physician Albert Wu.
Wu said this system also offers a glimpse into a new era of medical electronics. Since their introduction in the 1960s, electronic health records have reshaped the way doctors document clinical information, but decades later, these systems primarily serve as “an electronic notepad,” he added. With a series of machine learning projects on the way, from both Johns Hopkins and other groups, Saria said using electronic records in new ways could transform healthcare delivery, give doctors an extra pair of eyes and ears — and give them the opportunity to learn more. help make better decisions .
It’s a tempting vision, but one in which Saria, as CEO of the company developing TREWS, has a financial stake. This view also eliminates the difficulties of implementing new medical technology: providers may be reluctant to trust machine learning tools, and these systems may not work as well outside of controlled research environments. Electronic health records also pose many existing problems, from cluttering healthcare providers with administrative work to endangering patient safety due to software problems.
Still, Saria is optimistic. “The technology exists, the data is there,” she said. “We really need high-quality healthcare augmentation tools that allow healthcare providers to do more with less.”
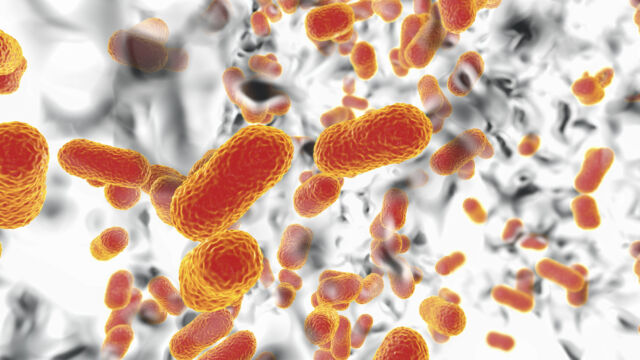
Currently, there is no single test for sepsis, so health care providers must make their diagnoses by reviewing a patient’s medical history, performing a physical exam, performing tests, and relying on their own clinical impressions. Given this complexity, over the past decade, physicians have increasingly used electronic health records to diagnose sepsis, usually using rules-based criteria — if this, then that.
One such example, known as the SIRS criteria, says that a patient is at risk for sepsis if two of the four clinical signs – body temperature, heart rate, respiratory rate, white blood cell count – are abnormal. This broadness, while useful for detecting the different ways sepsis can present itself, causes numerous false positives. Take a patient with a broken arm. “An automated system might say, ‘Hey look, fast heartbeat, fast breathing.’ It could give a warning,” said Cyrus Shariat, an ICU physician at Washington Hospital in California. The patient almost certainly does not have sepsis, but would raise the alarm nonetheless.
These alerts also appear on carriers’ computer screens as a pop-up, forcing them to stop whatever they are doing to respond. So while these rules-based systems occasionally reduce mortality, there is a risk of alert fatigue, with health professionals starting to ignore the stream of irritating memories. According to M. Michael Shabot, a trauma surgeon and former clinical chief of Memorial Hermann Health System, “It’s like having a constant fire alarm going off. You tend to be insensitive. You don’t pay attention.”
Electronic records are already not particularly popular among doctors. In a 2018 survey, 71 percent of physicians said records are a major contributor to burnout and 69 percent said they take precious time away from patients. Another 2016 study found that for every hour spent on patient care, doctors are required to spend an additional two hours on electronic health records and desk work. James Adams, chair of the department of emergency medicine at Northwestern University, called electronic health records an “overloaded swamp of information.”