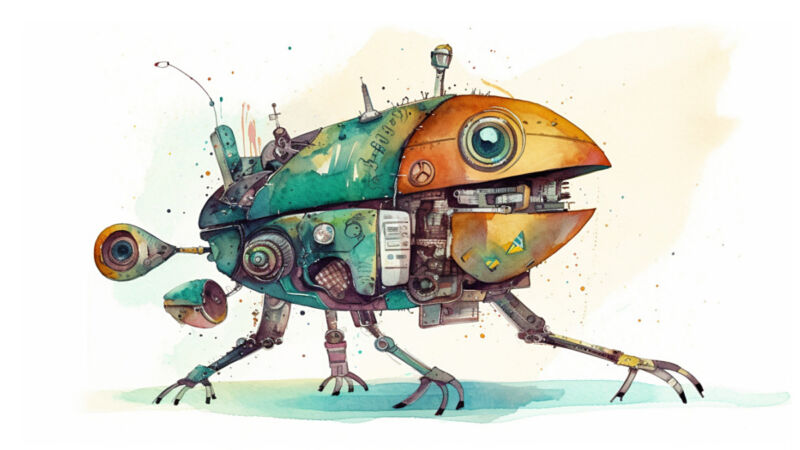
Half way through the journey
Since the launch of OpenAI’s GPT-4 API to beta testers last month, a loose group of developers has been experimenting with creating agent-like (“agentic”) implementations of the AI model that attempt to perform multi-step tasks with as little human intervention as possible. . These self-built scripts can iterate, iterate, and run new instances of an AI model as needed.
In particular, two experimental open source projects have garnered a lot of attention on social media, especially among those who hype AI Projects Relentless: Auto-GPT, Made By Toran Bruce Richardsand BabyAGI, made by Johei Nakajima.
Wat are they doing? well, now, not very. They need a lot of human input and handholds along the way, so they are not yet as autonomous as promised. But they represent early steps toward more complex AI models that may be more capable than a single AI model working alone.
“Achieve any goal you set independently”
Richards bills his script as “an experimental open source application that demonstrates the capabilities of the GPT-4 language model.” The script “chains LLM ‘thoughts’ together to autonomously accomplish any goal you set.”
In effect, Auto-GPT takes output from GPT-4 and feeds it back to itself with a makeshift external memory so it can repeat a task further, correct errors, or suggest improvements. Ideally, such a script could serve as an AI assistant that could perform any digital task on its own.
To test these claims, we ran Auto-GPT (a Python script) locally on a Windows machine. When you launch it, it asks for a name for your AI agent, a description of its role, and a list of five goals it’s trying to accomplish. During setup, you need to provide an OpenAI API key and a Google search API key. By default, when running, Auto-GPT asks for permission to execute every step it generates, though it also includes a fully automatic mode if you’re feeling adventurous.
If instructed to do something like “Buy a vintage pair of Air Jordans”, Auto-GPT will develop a multi-step plan and attempt to execute it. For example, it can search for shoe sellers and then search for a specific pair that meets your criteria. But then it stops because it can’t actually buy anything – right now. If linked to a suitable purchasing API, that could be possible.
If you want to get a taste of what Auto-GPT itself does, someone has made a web-based version called AgentGPT that works in a similar way.
Richards has been very open about his goal with Auto-GPT: to develop a form of AGI (artificial general intelligence). In AI, “general intelligence” typically refers to the still-hypothetical ability of an AI system to perform a wide variety of tasks and solve problems that have not been specifically programmed or trained for.
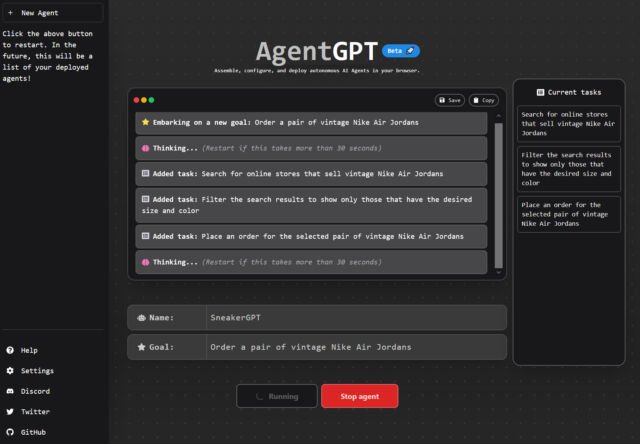
Ars Technica
Like a reasonably intelligent human being, a system with general intelligence should be able to adapt to new situations and learn from experience, rather than just following a set of predefined rules or patterns. This is in contrast to systems with narrow or specialized intelligence (sometimes called “narrow AI”), which are designed to perform specific tasks or operate within a limited number of contexts.
Meanwhile, BabyAGI (which gets its name from an ambitious goal to work on Artificial General Intelligence) works in a similar way to Auto-GPT, but with a different task-oriented flavor. You can try a version of it on the web at a not-so-humble site called “God Mode.”
Nakajima, the creator of BabyAGI, tells us he was inspired to create his script after witnessing the “HustleGPT” movement in March, which sought to use GPT-4 to automatically build businesses as a kind of AI co-founder, so to speak. “It made me curious if I could build a full AI founder,” says Nakajima.
Why Auto-GPT and BabyAGI are not AGI compliant is due to the limitations of GPT-4 itself. While impressive as a transformer and analyzer of text, GPT-4 still feels limited to a narrow range of interpretive intelligence, despite some claims that Microsoft has seen “sparks” of AGI-like behavior in the model. In fact, the limited usefulness of tools such as Auto-GPT may serve as the most powerful evidence yet of the current limitations of large language models. However, that doesn’t mean those limitations won’t eventually be overcome.
Also the issue of fabrications – when LLMs just make things up – may prove to be a major limitation to the usefulness of these agent-like assistants. For example, in a Twitter thread, someone used Auto-GPT to convert generate a report about companies that produce waterproof shoes by searching the internet and looking at reviews of each company’s products. GPT-4 may have “hallucinated” ratings, products, or even entire companies at every step of the process that weighed into the analysis.
When asked about a useful application of BabyAGI, Nakajima couldn’t think of any substantive examples other than “Do Anything Machine”, a project by Garrett Scott that aims to create a self-executing to-do list, which is currently in development. To be fair, the BabyAGI project is only about a week old. “It’s more of an introduction to a framework/approach, and the most exciting thing is what people are build on this idea,” he says.