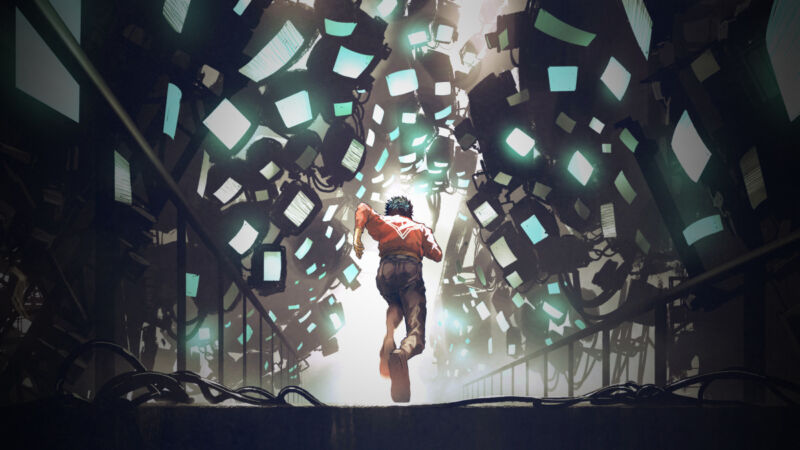
It's been a hugely busy week in AI news thanks to OpenAI, including a controversial blog post from CEO Sam Altman, the widespread rollout of Advanced Voice Mode, rumors of 5GW data centers, major personnel changes and dramatic restructuring plans.
But the rest of the AI world is not marching to the same rhythm, doing its own thing and coming up with new AI models and research by the minute. Here's a look at other notable AI news from the past week.
Google Gemini updates
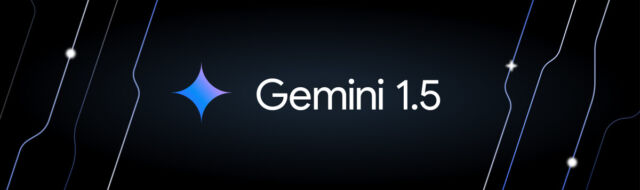
On Tuesday, Google announced updates to its Gemini model lineup, including the release of two new production-ready models that build on previous releases: Gemini-1.5-Pro-002 and Gemini-1.5-Flash-002. The company reported improvements in overall quality, with notable gains in math, long context processing and vision tasks. Google claims a 7 percent performance improvement on the MMLU-Pro benchmark and a 20 percent improvement in math-related tasks. But as you know, if you've been reading Ars Technica for a while, AI benchmarks are generally not as useful as we would like.
Along with model upgrades, Google introduced significant price reductions for Gemini 1.5 Pro, reducing input token fees by 64 percent and output token fees by 52 percent for prompts under 128,000 tokens. As AI researcher Simon Willison noted on his blog: “For comparison, GPT-4o currently costs $5/[million tokens] input and $15/m output and Claude 3.5 Sonnet is $3/m input and $15/m output. Gemini 1.5 Pro was already the cheapest of the frontier models and now it is even cheaper.”
Google has also increased rate limits, with Gemini 1.5 Flash now supporting 2,000 requests per minute and Gemini 1.5 Pro handling 1,000 requests per minute. Google reports that the latest models offer twice the execution speed and three times lower latency compared to previous versions. These changes can make it easier and more cost-effective for developers to build applications with Gemini than before.
Meta launches Llama 3.2
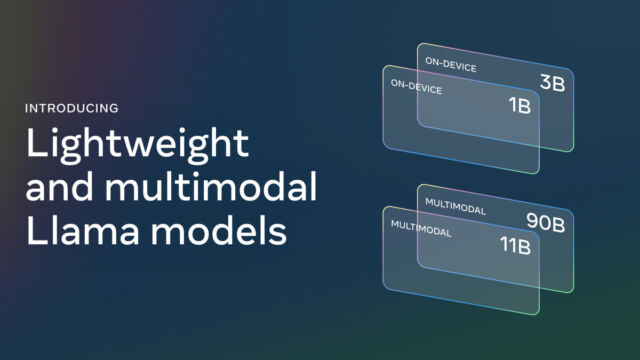
On Wednesday, Meta announced the release of Llama 3.2, a major update to its open-weight AI models that we've covered extensively in the past. The new release includes vision-capable large language models (LLMs) in parameter sizes of 11 billion and 90 billion, as well as lightweight text-only models of 1B and 3B parameters designed for edge and mobile devices. Meta claims that the vision models are competitive with leading closed-source models in image recognition and visual understanding, while the smaller models are said to outperform similarly sized competitors on several text-based tasks.
Willison did some experiments with some of the smaller 3.2 models and reported impressive results for the size of the models. AI researcher Ethan Mollick showed how he ran Llama 3.2 on his iPhone using an app called PocketPal.
Meta also introduced the first official “Llama Stack” distributions, created to simplify development and deployment in various environments. As with previous releases, Meta makes the models available for free download, with licensing restrictions. The new models support long context windows of up to 128,000 tokens.
Google's AlphaChip AI accelerates chip design
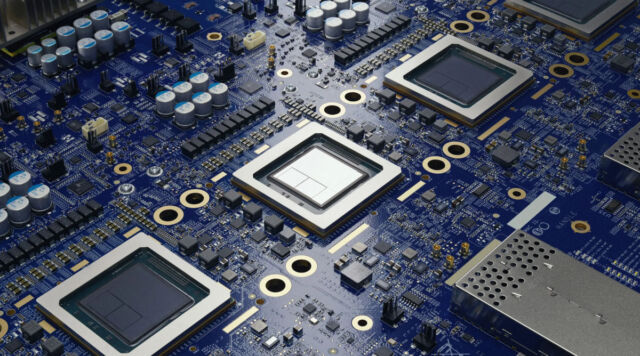
On Thursday, Google DeepMind announced what appears to be a significant advancement in its AI-powered electronic chip design, AlphaChip. It started as a research project in 2020 and is now a reinforcement learning method for chip layout design. Google has reportedly used AlphaChip to create “superhuman chip layouts” in the last three generations of its Tensor Processing Units (TPUs), which are chips similar to GPUs and designed to accelerate AI operations. Google claims that AlphaChip can generate high-quality chip layouts in hours, compared to weeks or months of human effort. (Nvidia has also reportedly used AI to help design its chips.)
Notably, Google has also released an AlphaChip pre-trained checkpoint on GitHub, where the model weights are shared with the public. The company reported that AlphaChip's impact already extends beyond Google, with chip design companies such as MediaTek adopting and building on the technology for their chips. According to Google, AlphaChip has led to a new line of research in AI for chip design, potentially optimizing every stage of the chip design cycle, from computer architecture to manufacturing.
That wasn't all that happened, but these are some major highlights. With the AI industry showing no signs of slowing down at the moment, we'll see how next week goes.